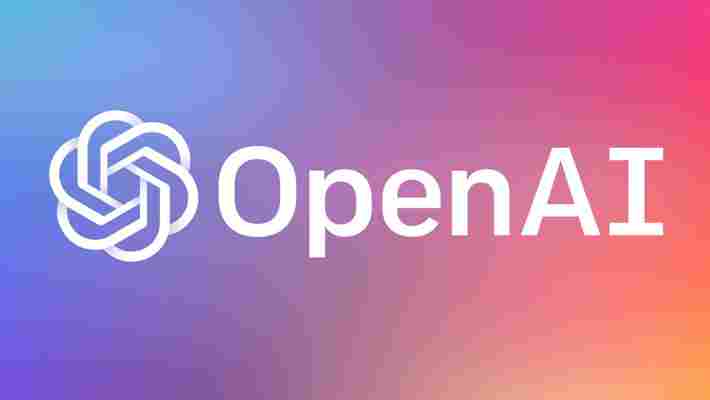
Why the nonprofit OpenAI made GPT-3 a commercial product
On March 16,2022 by Tom RoutleySo you like our media brand Neural? You should join our Neural event track at TNW2020 , where you’ll hear how artificial intelligence is transforming industries and businesses.
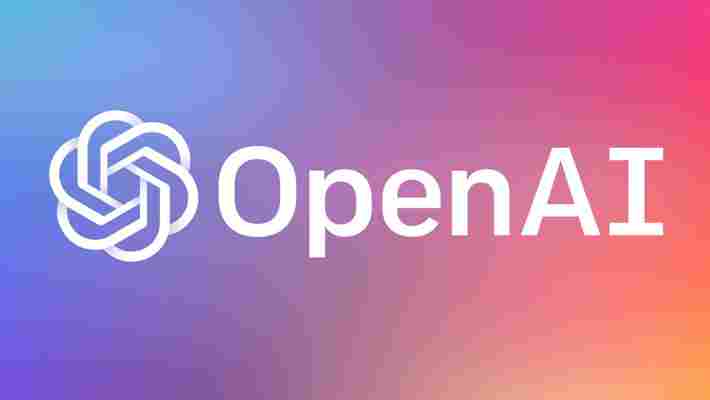
A program that can automate website development. A bot that writes letters on behalf of nature. An AI-written blog that trended on Hacker News. Those are just some of the recent stories written about GPT-3, the latest contraption of artificial intelligence research lab OpenAI. GPT-3 is the largest language model ever made, and it has triggered many discussions over how AI will soon transform many industries.
But what has been less discussed is how GPT-3 has transformed OpenAI itself. In the process of creating the most successful natural language processing system ever created, OpenAI has gradually morphed from a nonprofit AI lab to a company that sells AI services.
The lab is in a precarious position, torn between conflicting goals: developing profitable AI services and pursuing human-level AI for the benefit of all. And hanging in the balance is the very mission for which OpenAI was founded.
The change in OpenAI’s structure
In March 2019, OpenAI announced that it would be transitioning from a non-profit lab to a “capped-profit” company. This opened the way for funding from investors and large tech companies, with the caveat that their returns will be capped at 100x their investment (talk about capped!).
But why the structural change? In a post, the company announced that the move was meant to “rapidly increase our investments in compute and talent while including checks and balances to actualize our mission.”
The key phrase here is “compute and talent.”
Talent and compute costs are two of the key challenges of AI research. The talent pool for the kind of research OpenAI does is very small. And given the growing interest in commercial AI, there is fierce competition between large tech companies to acquire AI researchers for their own projects. This has triggered an arms race between tech giants, with each offering higher salaries and perks to attract AI researchers.
Google and Facebook have managed to snatch Geoffrey Hinton and Yann LeCun, two of the three pioneers of deep learning. Ian Goodfellow, a well-respected AI researcher and the inventor of generative adversarial networks (GAN), works at Apple. Andrej Karpathy, another AI genius, works at Tesla.
There is still ample interest in academic and scientific research, but with most AI talent being drawn to companies who can dish out stellar salaries , nonprofit AI labs are finding it harder to fill their ranks, unless they can match those salaries. According to a New York Times piece published in 2018, some of OpenAI’s researchers were making more than $1 million a year. DeepMind, another AI research lab, reported paying more than $483 million to its 700 employees in 2018.
Further increasing the cost of AI research is the computational requirements of artificial neural networks , the main component of deep learning algorithms. Before they can perform their tasks, neural networks must be trained on many examples, a process that requires expensive compute resources. In the past few years, OpenAI has engaged in several very costly AI projects, including a robot hand that solves Rubik’s cube, a gaming bot that beat the champions of Dota 2, and a group of AI agents that played hide-and-seek 500 million times.
According to one estimate, training GPT-3 would cost at least $4.6 million . And to be clear, training deep learning models is not a clean, one-shot process. There’s a lot of trial and error and hyperparameter tuning that would probably increase the cost several-fold.
OpenAI is not the first AI research lab to adopt a commercial model. Facing similar problems, DeepMind accepted a $650-million acquisition proposal from Google in 2014.
The change in OpenAI’s leadership
OpenAI started marketing investors under the leadership Sam Altman, one of the co-founders of the organization, who stepped down from his role as president of the acclaimed startup accelerator Y Combinator to become the CEO at OpenAI.
Before Altman, Greg Brockman was the face of the organization. Brockman, co-founder and CTO of OpenAI, is a seasoned scientist and engineer.
But in the tech investment space, reputation and product management skills are much more valued than scientific genius. And Altman is exactly the kind of person investors trust with their money. During his tenure at Y Combinator, he helped launch many successful companies including Airbnb and Dropbox.
In an interview with TechCrunch in May 2019, Altman said, “We have never made any revenue. We have no current plans to make revenue. We have no idea how we may one day generate revenue.”
But this didn’t detract investors from pouring money into OpenAI. In July Microsoft invested $1 billion in the company, knowing that Altman would somehow find a way to make the investment profitable.
The change in OpenAI’s mission
But there’s a fundamental conflict between the nature of tech investment firms and scientific research labs such as OpenAI.
OpenAI’s stated mission is to ensure that artificial general intelligence (AGI) “benefits all of humanity, primarily by attempting to build safe AGI and share the benefits with the world.”
But AGI is a lofty goal that is at least decades away by expert estimates. And tech investors are not known for their decades-long patience. They grow tired if they don’t get returns on their investment in a matter of years (just look at how the famed Boston Dynamics has been changing hands between investors in the past years despite posting viral YouTube videos of its robots).
How will OpenAI strike the right balance between AGI research and keeping its funders satisfied?
“OpenAI is producing a sequence of increasingly powerful AI technologies, which requires a lot of capital for computational power. The most obvious way to cover costs is to build a product, but that would mean changing our focus [emphasis mine]. Instead, we intend to license some of our pre-AGI technologies, with Microsoft becoming our preferred partner for commercializing them,” OpenAI wrote in the blog post that announced the Microsoft investment.
But there are clear signs that OpenAI is becoming—at least in part—a product company.
The commercial release of GPT-3
In May 2020, Microsoft declared creating one the world top-five supercomputers “in collaboration with and exclusively for OpenAI.” So, Microsoft tapped into OpenAI’s talent to create what Altman described as “our dream system.” The supercomputer will help OpenAI train its deep learning models, but it will also serve other customers of Microsoft’s Azure cloud computing platform.
Less than two weeks later, the first version of the GPT-3 paper was published on the arXiv preprint server. Unlike its predecessor GPT-2 , GPT-3 will not be released to the public. Instead, OpenAI has opted for a commercialized release, where developers can purchase access to GPT-3 through an application programming interface (API).
The OpenAI API announcement was made on June 11, though some developers were given early access to the technology.
This makes GPT-3 oddly similar to Microsoft’s Cognitive Services, a black-box cloud-based AI platform that gives developers API access to computer vision , natural language processing, and other AI functionality without providing the actual details of the model working behind the scenes.
This will at least help OpenAI return some of the investment Microsoft has made in the company. Microsoft also stands to gain a lot from the partnership, as it will probably have a deeper access to the technology and will be able to integrate it with its products such as Bing, Office 365, Outlookom, and Teams.
The commercial release of GPT-3 brings OpenAI one step closer to becoming an AI product company. And that’s one step away from nonprofit, scientific AI research.
Downplaying AI warnings
When they developed GPT-2, the OpenAI team decided not to release the AI to the public due to concerns about “malicious applications of the technology,” such as spreading spam and fake news. Instead, they adopted a phased approach, releasing smaller versions of the AI model and evaluating the results before making a larger model public.
While I argued at the time that a well-performing language model is not enough to create a fake news onslaught , I also supported the general idea of pausing and reflecting about the ramifications of technology before releasing it.
GPT-3 is three orders of magnitude larger than GPT-2. One of the key problems in deep learning language models is memory span. The AI starts to lose coherence as the text it generates becomes longer. Experiments have shown that larger neural networks in general have longer memory spans, which means that the potential of misuse in GPT-3 is much stronger than GPT-2.
This time, however, OpenAI didn’t make a lot of noise about GPT-3 becoming weaponized to create spam-bots and fake news generators. In contrast, OpenAI executives tried to downplay the warnings about the GPT-3. In July, Sam Altman dismissed the “GPT-3 hype” in a tweet.
Altman’s comments are mostly true, because AI still has a ways to go before it reaches human-level intelligence. Many experiments with GPT-3 show that despite the fascinating advances, the language model still struggles with some of the basic tasks that characterize intelligence.
But nonetheless, Altman’s comments have the hallmark of company executives reassuring investors that everything is under control.
OpenAI as a product company
Since its release, GPT-3 has been very well received by the tech community. Many developers and entrepreneurs have posted tweets of GPT-3 generating poems, memes, tweets, and website mockups.
One developer even managed to use GPT-3 to generate Python code for deep learning models.
Many of these posts are just amusing experiments. GPT-3 is not likely to take away any jobs soon. AI researchers and scientists have pointed out that the deep learning model is clearly not capable to tackle the kind of abstract cognitive problems that humans solve easily.
But GPT-3 has distinct benefits and potentially presents a tipping point in the business of AI. One of the key limits of deep learning systems is that they are narrow AI systems . They perform well on specific tasks but are poor at generalizing to other domains. To create a new deep learning application, you must either train a model from scratch or use transfer learning to finetune the parameters a pretrained model for a new task.
This limitation has stunted the deployment of AI services as platforms. While GPT-3 is still in the realm of narrow AI, it has proven to perform zero-shot learning on many tasks. This means that you can adapt it to many new applications without retuning its parameters.
This capability has already spawned many ideas for using the AI model to create new services. Debuildo is a company that uses GPT-3 to create web applications.
Augrented, a company that helps tenants research prospective landlords, is exploring ways to use GPT-3 to summarize legal notices or other sources in plain English to help tenants defend their rights.
And OthersideAI is using GPT-3 to provide creativity tools to users.
GPT-3 might eventually become a new platform on top of which a new crop of businesses and ecosystems will be created. This will be a success for Altman, but it will further draw OpenAI into the realm of becoming a product/services company. This is very different from releasing an open-source AI model and letting developers do what they want with it.
OpenAI must now satisfy customers, scale its infrastructure, deal with compliance issues, and much more. And with its AI model becoming the bread and butter of newly spawned startups, OpenAI will also have to deal with some of the specific challenges of running a deep learning business . OpenAI will still have to handle problems such as removing harmful biases and dealing with model decay. Those are all costly tasks, especially when dealing with a 175-billion-parameter deep learning model.
And OpenAI still has to figure out how to do all these things while also remaining profitable.
Although Altman is a very successful entrepreneur, he won’t be able to run the operations of the company alone. As OpenAI further wades into the realm of product management, it will need even more help from Microsoft.
OpenAI already relies on Microsoft’s cloud infrastructure to train and run its models. But it may soon need the tech giant’s help to deal with legalities, customer support, privacy and security, product scaling, and much more.
The future of OpenAI
OpenAI’s story depicts the challenges of scientific AI research. For the moment, the popular belief is that bigger deep learning models will lead to more advanced AI systems. This means AI research labs will need a lot of money to acquire talent and train their increasingly bigger deep learning models.
The only organizations willing to dole out such amounts of cash for the moment are large tech companies. But tech investors also expect returns on investment, forcing research labs to use part of their resources to create profitable products. In time, the larger company might completely absorb the lab into its own commercial goals.
We’ve already seen this play out after a fashion after Google acquired DeepMind. The AI lab had to split its resources between AGI research and an “applied AI” division that works on creating profitable products. But the company has yet to even out the costs it is incurring for its owners.
As for OpenAI, the company is now walking a fine line. The more the company becomes enmeshed with commercializing its AI services, the harder it will be for it to stick to its original mission. Will it adhere to the transparent, open-source nature of scientific research on human-level AI, or will it gravitate toward the wall-gardened approach of commercial entities, closely guarding its research as company secrets and intellectual property? Will it uphold its “ primary fiduciary duty to humanity ,” or will the satisfaction of investors (and potential future owners) become its main focus?
Time will tell.
This article was originally published by Ben Dickson on TechTalks , a publication that examines trends in technology, how they affect the way we live and do business, and the problems they solve. But we also discuss the evil side of technology, the darker implications of new tech and what we need to look out for. You can read the original article here .
Article Recommendations
Latest articles
Popular Articles
Archives
- November 2024
- October 2024
- September 2024
- August 2024
- July 2024
- June 2024
- May 2024
- April 2024
- March 2024
- February 2024
- January 2024
- December 2023
- November 2023
- October 2023
- September 2023
- August 2023
- July 2023
- June 2023
- May 2023
- April 2023
- March 2023
- February 2023
- January 2023
- December 2022
- November 2022
- October 2022
- September 2022
- August 2022
- July 2022
- June 2022
- May 2022
- April 2022
- March 2022
- February 2022
- January 2022
- December 2021
- November 2021
- October 2021
- September 2021
- August 2021
- July 2021
- January 2021
Leave a Reply